Approximation Capabilities of Mixture of Experts Models
Mar 29, 2020
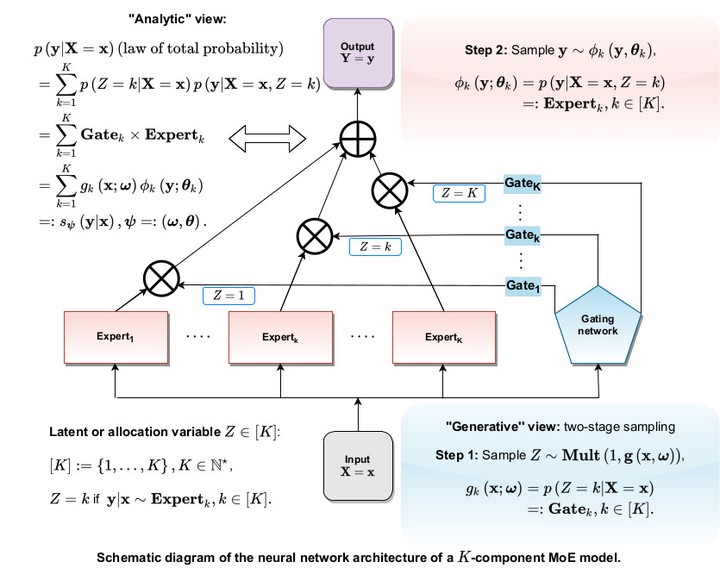
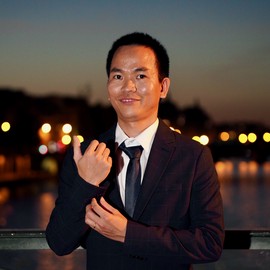
TrungTin Nguyen
Postdoctoral Research Fellow
A central theme of my research is data science at the intersection of statistical learning, machine learning and optimization.
Publications
Approximation of probability density functions via location-scale finite mixtures in Lebesgue spaces
The class of location-scale finite mixtures is of enduring interest both from applied and theoretical perspectives of probability and …
Approximations of conditional probability density functions in Lebesgue spaces via mixture of experts models
Mixture of experts (MoE) models are widely applied for conditional probability density estimation problems. We demonstrate the richness …
Approximation by finite mixtures of continuous density functions that vanish at infinity
Given sufficiently many components, it is often cited that finite mixture models can approximate any other probability density function (PDF) to an arbitrary degree of accuracy. Unfortunately, the nature of this approximation result is often left unclear. We prove that finite mixture models constructed from pdfs in $C_0$ can be used to conduct approximation of various classes of approximands in a number of different modes. That is, we prove approximands in C0 can be uniformly approximated, approximands in $C_b$ can be uniformly approximated on compact sets, and approximands in Lp can be approximated with respect to the $L_p$, for $p\in [1,\infty)$. Furthermore, we also prove that measurable functions can be approximated, almost everywhere.
Talks
Model Selection and Approximation in High-dimensional Mixtures of Experts Models$:$ From Theory to Practice
Mixtures of experts (MoE) models are a ubiquitous tool for the analysis of heterogeneous data across many fields including statistics, …
Dec 14, 2021 1:30 PM — 6:00 PM
Laboratoire de Mathématiques Nicolas Oresme, Université de Caen Normandie, France